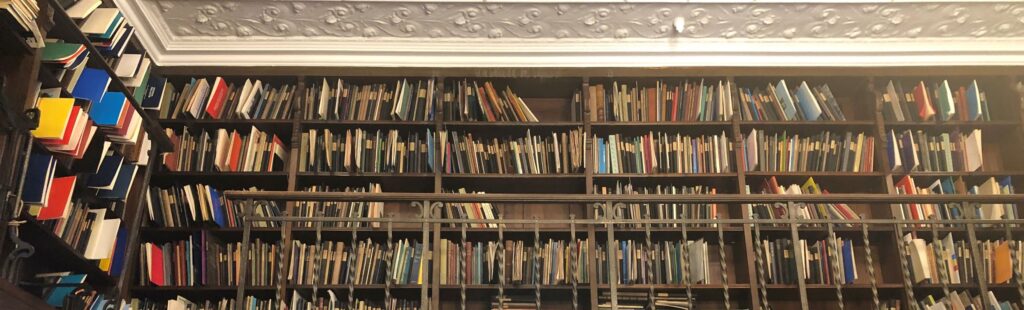
2022-01-23 / Updated 2022-01-27 / Ahn Louise
Variability Matters for Process Flow Cycle Time
If you have the responsibility for or is part of some process flow, you should know the following:
- Little’s law: [Work in process] = [Throughput] x [Cycle time]
- Queue time before a process depends on variability in arrival and process time, utilisation (non-linearly) and average process time. This means that when you get close to your capacity limit (when utilisation is high), your queue time, and hence your cycle time will increase wildly.
- Variability propagates through the flow. This means that variability in the beginning of the flow has a larger impact on the total cycle time than variability in later processes.
- Many small interruptions cause lower variability than few large interruptions.
So you need to understand that your capacity is not determined by average processing time alone. And that large interruptions can cause extreme cycle times.
An example which I have witnessed myself, was when a queue in a Quality Control function exploded due to Christmas vacation. This led to cycle times far beyond the lead time target. Because the function was manned to work at relatively high utilisation, it took several weeks to get the backlog and the cycle time back in control. The situation was unpleasant for the QC function returning from Christmas to a high workload, and it caused issues in several parts of the supply chain. Now, this was caused by a reduction in capacity, but the outcome would have been the same if a sudden surge in demand had occurred.
How to Meet Lead Time Requirements
Ensure Sufficient Capacity
If you want to be in control of your cycle time, you need have sufficient capacity. This may be based on your experience with the processes, if there are no big changes to the underlying patterns. But if you really want to understand what level of output your process is capable of delivering with a given capacity, you need to understand both averages and variability in demand and process times.
Formulas to approximate performance exist for simple linear flows. With more complex flows, simulation could be a way of gaining an idea of the possible performance. You can find the formulas in this article and download an Excel sheet where the formulas are used to calculate cycle time and work-in-process for a simple workflow.
Reduce Variability
If you find that your capacity is insufficient to meet your lead time requirements, you may of course increase your capacity. But maybe there is a cheaper alternative. What if you could instead reduce the variability? Of course, not all variability is within your control, but chances are that a large part of the variability comes from the way you run your processes, including different supporting processes such as maintenance.
Hi Ahn Louise, great point!
In my experience, if CONWIP is applied (see FP) it is possible to obtain both a decrease in cycle time and a containment of flow variability, so that throughput decreases but only slightly as the WIP decreases.
I have verified this several times with my FP math-based analytical simulator.
Sandro Rizzoli
Bologna (Italy)
I definitely also need to experiment with CONWIP job release to see how it impacts cycle time and throughput. Thanks for the input 🙂
Best regards, Ahn Louise
I also use Kanban in my simulations (I mean Kanban as “WIP buffer limitation for each phase”). Kanban, in the presence of variability, is often less performing than CONWIP, since it significantly reduces cycle time, but also significantly reduces throughput.